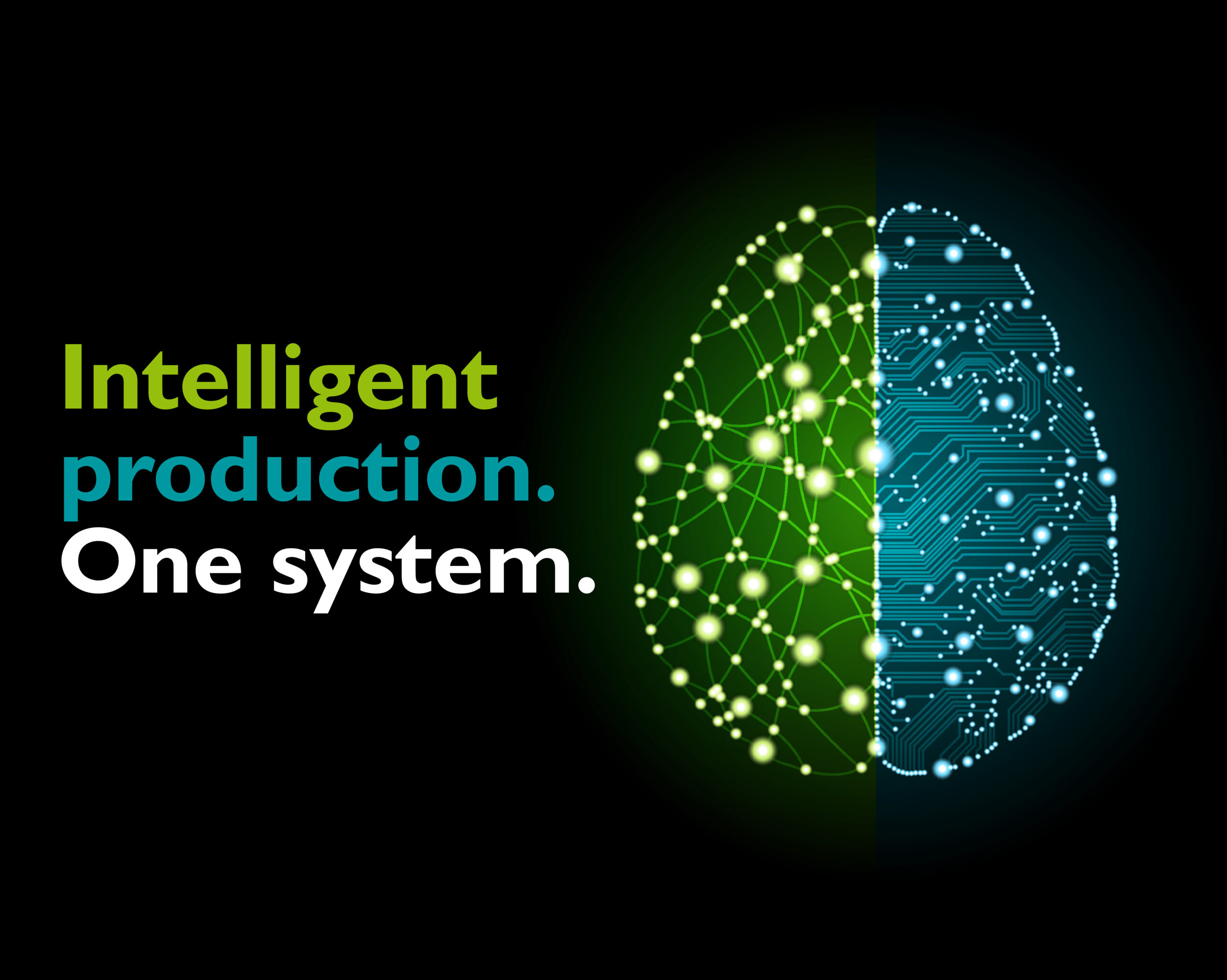
The increasing use of artificial intelligence (AI) in industrial applications can solve highly complex challenges. Rising data volumes resulting from increasingly complex IoT sensors and actuators require machines and processes that can independently gain insights and optimize processes. That’s why more and more companies are harnessing the power of artificial intelligence and developing innovative approaches to simplify people’s working lives with the help of AI, Big Data, and digital assistants.
Intelligent machines take over tasks that are difficult, dangerous, or impossible for humans due to the enormous amounts of data, thus creating capacities for tasks that require human awareness. Especially in the industrial sector, artificial intelligence is already being used by many companies to develop individual concepts.
In the following article, we would like to show examples of how such solutions can look in various application areas.
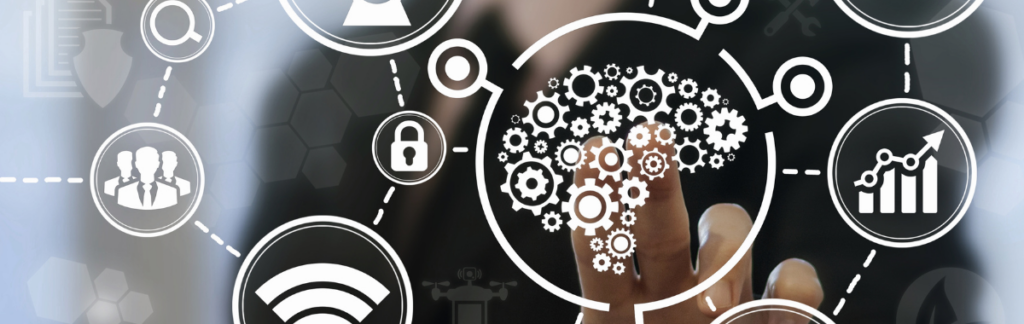
The potential of artificial intelligence is enormous: driverless transport systems independently find their way through factory halls; plants adjust themselves during operation and optimize themselves – to name just a few examples. The term “artificial intelligence,” which is not clearly defined, encompasses many designations. Put simply, computer programs can both mimic human intelligence and make up their own rules to learn on their own through experience. In this way, the system can independently optimize itself to complete tasks without human assistance. Buzzwords that fall under “AI” include machine learning (ML) and deep learning (DL). Machine learning is about tackling a problem based on past experience. For example, based on datasets and algorithms that must be generated by the user, predictive maintenance applications can be developed to predict future events. Anomalies are independently compared with the data sets, for example, to calculate the service life of the plant.
Deep learning is a subfield of machine learning that uses neural networks. Neural networks are structures that mimic the human brain and thus learn unsupervised from unstructured and unlabeled data. Deep learning algorithms consequently improve and acquire new capabilities without human intervention. Unlike machine learning, a deep learning algorithm no longer needs to be told what features to look for. For a comprehensible presentation of the use cases, both methods are contrasted with the goal of developing an image processing and resulting object recognition.
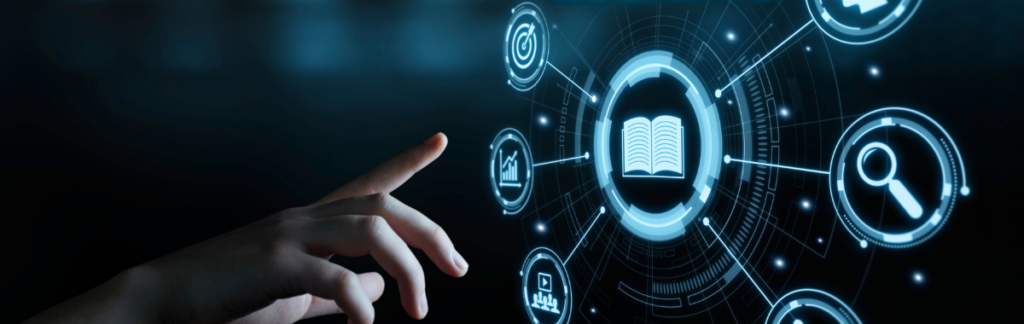
Solve more tasks with an algorithmic chain
In classical image processing, based on manual training of the model, there are numerous available and reliable algorithms that have proven to be efficient and transparent. Thus, it is explained to the user how the result is obtained. The disadvantage of such an approach is that the rather inflexible procedure must be tailored to specific tasks. It may be necessary to model each inspection task in a complex way so that specific objects can be classified. Deep-learning-based methods, on the other hand, enable high quality object recognition. Compared to the classical method, a higher accuracy and error tolerance can be guaranteed. Another advantage results from the fact that the same algorithmic chain can be used to solve other problems. Consequently, a different network can classify a different object. Neural networks can thus be retrained. However, enormous computing power is required to perform such a procedure.
While artificial intelligence has long since found its way into the consumer sector, real applications are now also coming into use in the industrial environment. Employees use voice and image recognition via their smartphones on a daily basis, office buildings are fully autonomous and react to any external influences such as heat, cold, light or wind. These and far more complex sub-areas of AI are used to continuously optimize processes and increase the efficiency of machines and systems accordingly.
Proven in quality control
One example of the use of AI in industry is the detection of quality defects, for example in the food industry. A chocolate bar is detected by several cameras and compared with good or bad images using a database that was previously created manually. This is a classic application of machine learning.
Another example is the quality control of plastic injection molded parts for the automotive industry. Here, the focus is on absolute zero-defect tolerance, which is to be ensured by image processing. Another requirement is to develop a flexible process that can be used to analyze different parts. The AI specialists are using a deep learning approach. For this purpose, the object is classified in order to determine at which points an error occurs. In addition, the network is designed as a so-called anomaly detection. This makes it possible to detect whether and to what extent the bad parts deviate from the good parts. As an added value for the operator, the same inspection routine can be used for different parts. He also does not need AI knowledge to work with such systems. The system only needs to be set up once.
Implement processes in real time with powerful hardware
In the field of smart cities, there are many application examples for the sensible use of artificial intelligence. A networked, intelligent infrastructure offers great potential for optimizing processes using AI. In the context of smart infrastructure, this can range from the switching of traffic lights and lighting systems to traffic monitoring based on sensor systems for traffic optimization. In addition, parking space management is conceivable so that parking garages in large cities are better utilized and drivers do not have to spend a long time looking for a parking space. Vehicle-to-vehicle communication is also possible.
As with industrial applications, the scenarios described above result in the important requirement for compact and powerful hardware. Here, for example, an AI extension module can be used. These can accelerate applied processes and realizing them in real time. Thus, the user can develop an autonomous or cloud-based application, directly influence PLC variables, and interact with them. Based on artificial intelligence, the module evaluates the raw data from the sensors at the point of origin.
The overall structure of a corresponding application can look as follows: The controller including the AI extension module is installed at the field level – i.e., on site in the city – and connected there with the respective sensor technology. Data communication takes place either directly with the IT systems or via a cloud solution. A special feature of open control platforms is the connection of the control system to an industrial app store. New apps can simply be loaded onto the PLC from the digital marketplace, which is why the same hardware platform can be used for different use cases in an uncomplicated manner.
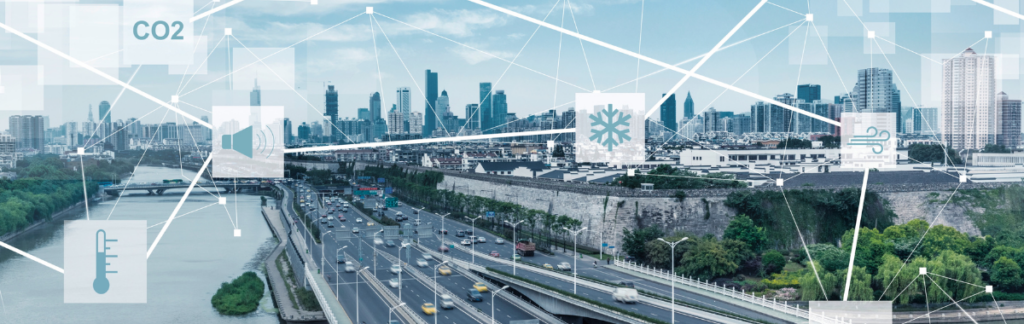
Conclusion
Through the increasing use of artificial intelligence, highly complex challenges in industrial applications can be solved individually. Use cases in quality control or smart cities in particular offer great potential for optimizing processes in this way and developing zero-defect tolerance. Thanks to compact, powerful hardware that can be connected directly to the controller, the raw data from the sensors can already be evaluated at the point of origin using machine learning or deep learning methods, resulting in the following benefits:
– More flexibility: The intelligence can handle unknown objects even without programming.
– More productivity: Machines react automatically and flexibly to situations that previously had to be handled manually. This reduces downtime and increases availability.
– More quality: Processes can be checked reliably and quickly by trained neural networks.
– More effectiveness: Problems in production are detected at an early stage, thus saving costs for rework or rejects.